Most industries are witnessing massive transformation since the evolution of Generative AI tools in the last few years. Extending its capability to provide information based on prompts, GenAI significantly saves a lot of time and enhances scalability of industries dissecting its intangible business benefits across various functions. It includes implementation of Large Language Models (LLMs), generative AI principles, and other AI algorithms as well.
All these make generative AI powerful as its in-built capabilities to deal with data patterns are truly incredible. Moreover, generative AI is used to generate information needed in the form of prompts. But the new evolution has its own set of challenges to deal with, especially when it’s providing answers about the questions asked.
The reason is, generative AI uses generalized LLM where the data may not be accurate or may be weeks or months old. It may not include the specific information related to an organization’s products or services. This clearly gives an understanding that the scope of generative AI is limited it may generate erroneous information at times.
What is Retrieval Augmented Generation (RAG)
To leverage generative AI’s limitations, Retrieval-Augmented Generation (RAG) whirls in, which fine tunes and optimizes the output of a generalized LLM. However, underlying model remains unchanged, ensuring targeted information is accurate and specific to the domain or industry. All the answers that RAG provides will be based on current trending data for the given prompts.
Generative AI developers came to know about RAG when “Retrieval-Augmented Generation for Knowledge-Intensive NLP Tasks,” authored by Patrick Lewis and a team at Facebook Research was published. Many prominent industry leaders and researchers began to encompass RAG innovation and believed that it can significantly boost the value of generative AI models.
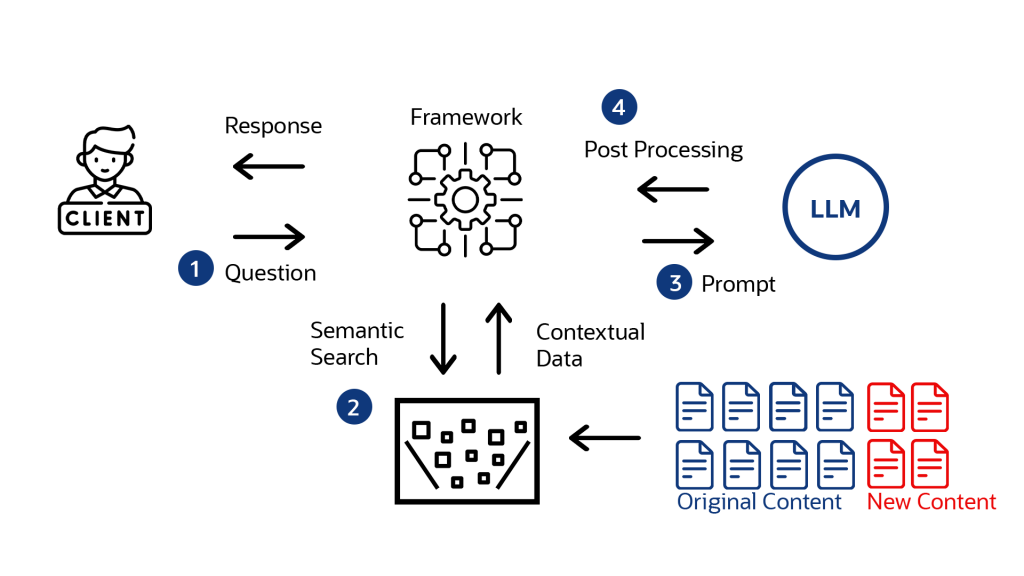
Retrieval-Augmented Generation – Where AI Excels at Multiplying Business Value
Consider an example of an IT industry that’s hosting a big tech event. The industry experts and people want to use chat to answer questions on whereabouts of the event including event location, thought leaders who voice out in the event, ticket pricing, topics that will be spoken etc. In this case, a generalized LLM gives its answers to some extent but it can’t really provide in depth information about the event. RAG ingests LLMs intelligence to access information from multiple sources such as data warehouses, databases, personas, and news feeds posted on company’s portfolio, thereby showing relevant information. Now, timely, accurate, and trending updates are provided with the help of RAG.
It’s clear that RAG is a new AI algorithm where AI’s quality is enhanced by enabling LLMs to tap into all the data sources without any retraining. Through this, RAG models build knowledge repositories and information is updated accordingly in AI so that information is more accurate, contextual, and timely. Implementation of RAG comes with integration of vector databases where they allow rapid coding of new data sets and it searches as per the data fed into LLM.
Demystifying RAG Process
RAG scans through structured databases, unstructured PDFs, other documents, blogs, press releases, news feeds of your social media platforms, and chat transcripts from recorded customer service sessions of your organization. All this dynamic data is stored in its knowledge library that is accessible to the generative AI. Now, the data in library is represented in the numerical form using embedded language model, a special algorithm designed to embrace RAG’s competence. Whatever the data is collected, it is stored in a vector database and the algorithm quickly searches and retrieves right contextual information.
When a specific prompt is fetched to Gen AI, the query gets transformed to a vector and the response is sent to the database. Thereby the information is retrieved from the database, correlating with the prompts that were sent. Here the contextual info and original prompt is pushed into LLM which generates a text response that is accurate.
When playing with generalized LLM, it must be trained before generating any information which is time-consuming. Hence the integration of RAG practice is essential for its fastness and accuracy. Any form of new data can be imbibed into embedded language model and translated into vectors on a progressive and continuous manner. Any misinformation generated by gen AI is easily corrected and fed into vector database, thus making RAG to be more timely, contextual, and accurate.
Core Model of RAG: Semantic Search
RAG is only one of the techniques that promises accuracy of LLM-based generative AI. One more practice is semantic search. Semantic search narrows the search and provides the right meaning of a query by using deep learning models for the specific words and phrases in the prompt. Though the traditional search focuses on keywords, semantic search dives deep into retrieving the meaning of questions and source documents. It utilizes these meanings and gives more accurate results. This makes semantic search to function as an integral part of Retrieval-Augmented Generation.
Leveraging the Use of RAG in Chat Applications
Many industries have deployed chatbots to handle customer queries but they don’t have high responsiveness and trained to provide finite answers. This is the time for business leaders to quickly move in to understand RAG capabilities as it can give responses that are outbound to the intent list. Every question comes with a specific context and users expect relevant and accurate answers. Hence, integration of RAG into chatbots delivers smooth and optimized customer experience.
Benefits of RAG
- Optimizes the quality of Gen AI’s responses.
- RAG has the access to information that’s timely and accurate.
- RAG’s knowledge repository functions as a holder of contextual data.
- The information sources can be identified quickly and incorrect information can be corrected or deleted from the system.
RAG Implementation Challenges
- As RAG unravelled in 2020, still AI developers are experimenting on how best they can bring this into real world by optimizing information retrieval mechanisms.
- Improving RAG practices in the organizations and understanding RAG.
- Cost concerns come into consideration as generative AI with RAG is more expensive.
- Using structured and unstructured data present in vector database is still in working phase.
- Continuous data feeding mechanism into RAG system is in experimentation phase.
- Setting up processes to handle inaccuracies and other misleading information as well as deleting/correcting from RAG system seem to be complex.
Implementation of Retrieval-Augmented Generation within Oracle
RAG being the futuristic technology, it’s proving to provide appropriate action based on contextual information. Touching the innovation edge, Oracle offers a wide variety of cloud-based AI services and OCI generative AI service runs on OCI while delivering robustness and enhancing security for your organization. Any of the customer data is not shared with LLM providers.
As an esteemed Oracle’s Go-To Partner providing End-to-end solutions for years, generative AI models are integrated into most of our Oracle applications. Developers who use OCI with embedded generative AI capabilities can deliver greater performance at competitive pricing. In the breathless execution of generative AI across industries and use cases, RAG technology makes any business to stand on innovation edge thereby leveraging its operational models. That’s where Infolob joins the chain to carve perfection for you through Oracle practice, capitalizing your business value.
For all queries, please write to: